Encoding Lightfields with Coordinate-based Neural Networks
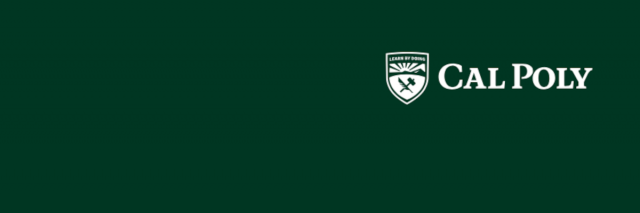
About the Project
Neural Lightfields
- Lightfields are a 4D structure consisting of sub aperture images arranged in a sphere around the viewer
- A Plenoptic camera, which simulates an array of individual cameras, is used to capture lightfields
- They contain data capable of recreating 3D scenes, say for virtual reality
- Neural Lightfields are created using a trained neural network
Coordinate-based Neural Networks
- Coordinate Based Neural Networks are used in Computer Vision tasks to encode and compress N-Dimensional structures
- Using a simple RGB image as an example:
- Input Vector à 3D Coordinate
- Output Vector à RGB Pixel Value
- On more complex media i.e. 5D Voxel grid, a network can provide high compression
- 5D Voxel Grid (+10Gb) à Trained weights (~5Mb)
- Model Weights are trained to encode a single N-Dimensional structure
- Every new example must be trained individually
- This is slow and not ideal for real time applications
Model Agnostic Meta Learning (MAML)
- Normally, starting weights for training a model are random
- If the targets of training are similar images for CBN, we can find starting weights that make training converge faster
Team
Samuel Cole, Computer Science
Dr. Jonathan Ventura, Computer Science
Showcase poster1.63 MB